AI Development: From Early Concepts to Modern Generative Tools
Written by Angelo Consorte | Published on November 28, 2024
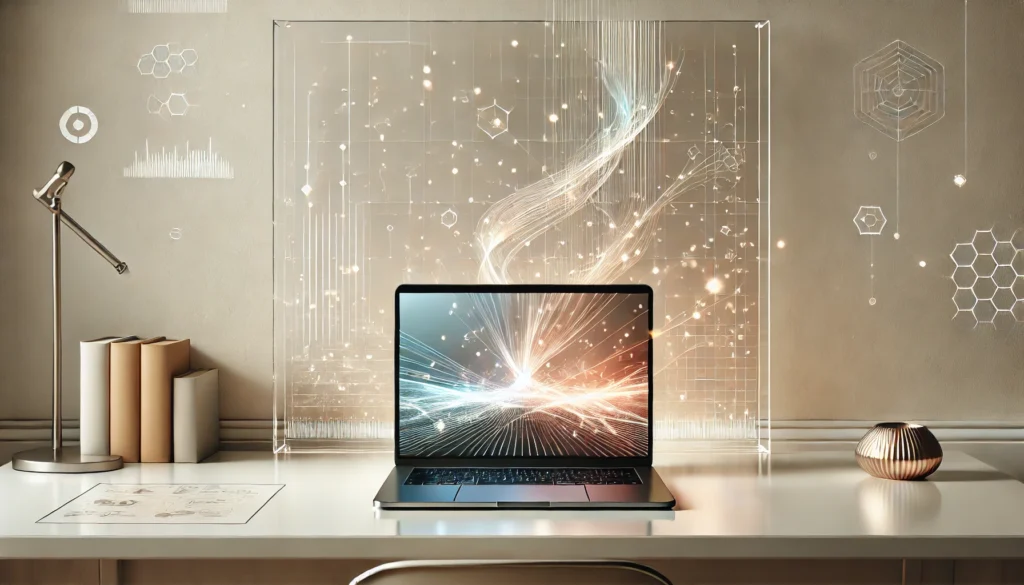
Introduction
Artificial Intelligence (AI) has become increasingly important, particularly in recent years. It is almost unimaginable not to hear or read the term “Artificial Intelligence” at least once within a week.
In late October and early November 2024, Apple began introducing its proprietary AI applications, branded as Apple Intelligence, across its devices.
Furthermore, in October of this year, OpenAI secured an unprecedented $6.6 billion in funding, which is expected to drive extensive research and development efforts, expand computational capabilities, and further its mission to advance the field of artificial intelligence.
According to a report by Bloomberg, the generative AI sector alone is projected to evolve into a $1.3 trillion market by 2032.
These developments suggest we are at the threshold of a transformative era, one with the potential to shape our future profoundly. But what exactly is AI? Why is this technology becoming so prevalent in our daily lives? What are its applications, and in what contexts does it find relevance?
History of AI
In the early 20th century, science fiction introduced the concept of artificial intelligence through iconic characters like the Tin Man from The Wizard of Oz. By the 1950s, this cultural groundwork had profoundly influenced a generation of scientists and mathematicians, embedding the concept of AI deeply into their intellectual and creative pursuits.
Alan Turing, a British polymath, was one of many from this generation who began exploring the mathematical foundations of artificial intelligence. In his 1950 paper Computing Machinery and Intelligence, he proposed that machines could solve problems and make decisions using information and reasoning, much like humans. However, Turing’s progress was limited by early computers’ inability to store commands and prohibitively high costs, which required proof of concept and influential support to advance research.
In 1956, the “Logic Theorist” became the first AI program ever developed. Created by Allen Newell, Cliff Shaw, and Herbert Simon, this work served as proof of Alan Turing’s early ideas on machine intelligence. The program mimicked human problem-solving and was presented at the Dartmouth Summer Research Project on Artificial Intelligence, where John McCarthy coined the term “artificial intelligence.”
Over the next few decades, as computing technology became faster, cheaper, and more accessible, significant advancements occurred in AI. Machine learning algorithms improved, John Hopfield and David Rumelhart popularized deep learning techniques allowing computers to learn from experience, and Edward Feigenbaum introduced expert systems mimicking human decision-making processes. Gradually, the obstacles that had seemed insurmountable in AI’s early days became less significant. The fundamental limitation of computer storage, which held back progress 30 years earlier, was no longer an issue.
Now, in the era of big data, vast amounts of information exceed human processing capacity. AI has proven valuable across numerous industries, leveraging massive datasets and computational power to learn efficiently.
With sufficient computational and storage capacity, AI is now accessible to a broader audience, enabling everyone to benefit from decades of research and innovation.
Definition
Artificial intelligence is essentially technology that enables machines and computers to mimic human behaviors like learning, solving problems, creating, and making decisions.
In everyday life, AI is everywhere. It helps unlock smartphones with facial recognition and corrects typing errors with autocorrect. Search engines like Google predict what you are looking for as you type, while social media apps like Instagram or TikTok display posts tailored to your interests.
- Facial recognition systems use computer vision and machine learning algorithms, often based on convolutional neural networks (CNNs), to analyze and compare facial features from an image or video to stored data.
- Autocorrect relies on natural language processing (NLP). It uses language models trained on large text datasets to predict and suggest the most likely word based on context and spelling patterns.
- Search engines use NLP, machine learning, and data mining to analyze previous search data, user behavior, and linguistic patterns. Algorithms like Google’s RankBrain evaluate context to provide real-time suggestions and refine results.
In 2024, most AI researchers and headlines are centered around advancements in generative AI—a technology capable of producing original text, images, and videos. To understand generative AI, it’s essential to grasp the foundational technologies on which it relies.
AI can be understood as a hierarchy of concepts:
- Artificial intelligence (machines that mimic human intelligence)
- Machine learning (AI systems that learn from historical data)
- Deep learning (ML models that mimic human brain functions)
- Generative AI (deep learning models that create original content)
- Deep learning (ML models that mimic human brain functions)
- Machine learning (AI systems that learn from historical data)
The evolution of these concepts is why we can now leverage advanced tools to enhance productivity in various areas of life.
AI tools like ChatGPT have brought this technology into the public spotlight, prompting questions about what makes today’s AI advancements different and what the future holds. The field has experienced cycles of progress and skepticism, known as the “seasons of AI.” However, this “AI summer” marks a new phase of sustained impact, rather than inflated expectations.
Currently, much of AI’s focus is on productivity. According to PricewaterhouseCoopers, the boost in productivity enabled by AI could add $6.6 trillion to the global economy by 2030.
AI has become integral to daily life, enhancing productivity and transforming industries with its ability to mimic human intelligence. From foundational technologies like machine learning and deep learning to advancements in generative AI, its evolution continues to shape the future. However, as AI grows, so does the hype, often blurring the line between true innovation and exaggeration—an issue worth exploring further.
AI in Context
The potential of AI is vast and exciting, yet the term “artificial intelligence” is often used loosely to encompass a wide range of technologies and approaches that mimic aspects of human intelligence. This broadness has made AI a prime target for marketing and hype, with companies frequently labeling systems as “AI” to attract attention, even when these systems lack genuine learning capabilities or advanced intelligence.
For instance, a simple chatbot that relies on basic keyword matching may be labeled as “AI” simply because it mimics conversational behavior, even though it lacks the characteristics of more complex AI models, such as learning from interactions or understanding context. This kind of misrepresentation fosters unrealistic expectations among users and undermines the credibility of AI as a transformative technology.
When tools are marketed as AI without possessing advanced capabilities, it becomes harder for consumers and businesses to discern genuine innovation from superficial features. This confusion can lead to misplaced investments, skepticism about AI’s potential, and missed opportunities to adopt tools that could truly enhance productivity and problem-solving.
Conclusion
Artificial intelligence has firmly established itself as a transformative force in modern society, driving innovation on different industries and reshaping the way we interact with technology. From its historical origins and foundational advancements to the latest breakthroughs in generative AI, the journey of AI reflects the pursuit of mimicking human intelligence to enhance efficiency, creativity, and decision-making.
However, with great potential comes the need for discernment. The continuous growth of AI, requires us to understand how to distinguish genuine advancements from overhyped claims. As we stand on the cusp of an AI-driven future, informed understanding and ethical innovation will be key to harnessing its true power while ensuring its benefits are accessible and meaningful for all.
References
- Bean, R. (2017, May 8). How big data is empowering AI and machine learning at scale. MIT Sloan Management Review. https://sloanreview.mit.edu/article/how-big-data-is-empowering-ai-and-machine-learning-at-scale/
- Smith, R. (2024, February 8). The Ai Explosion, explained. Duke Today. https://today.duke.edu/2024/02/ai-explosion-explained
- Bloomberg. (2023, June 1). Generative AI to become a $1.3 trillion market by 2032, research finds | press | Bloomberg LP. Bloomberg.com. https://www.bloomberg.com/company/press/generative-ai-to-become-a-1-3-trillion-market-by-2032-research-finds/
- Stryker, C., & Eda Kavlakoglu, E. (2024, October 25). What is Artificial Intelligence (AI)?. IBM. https://www.ibm.com/topics/artificial-intelligence
- Johnson, A. (2024, October 28). Apple Intelligence is out. The Verge. https://www.theverge.com/2024/10/28/24272995/apple-intelligence-now-available-ios-18-1-mac-ipad
- IV, A. P. (2024, October 4). OpenAI valued at $157 billion after closing $6.6 billion funding round. Forbes. https://www.forbes.com/sites/antoniopequenoiv/2024/10/02/openai-valued-at-157-billion-after-closing-66-billion-funding-round/
- Anyoha, R. (2020, April 23). The history of Artificial Intelligence. Science in the News. https://sitn.hms.harvard.edu/flash/2017/history-artificial-intelligence/
- TURING, A. M. (1950). I.—Computing Machinery and intelligence. Mind, LIX(236), 433–460. https://doi.org/10.1093/mind/lix.236.433